dqs.torch.distribution.DistributionLinear
PyTorch layer to represent a probability distribution as a cumulative probability distribution (CDF). See page predicting probability distribution for more detailed explanation. In this layer, a CDF is represented as a piece-wise linear function.
class DistributionLinear(
boundaries,
axis='target')
Parameters
Args |
Type |
Description |
---|---|---|
boundaries |
Tensor (float) |
One-dimensional tensor to specify the boundaries of the knots of the CDF. This parameter must be sorted in an increasing order. |
axis |
string |
Set axis = ‘target’ if you want to use distribution regression. If axis=’target’, the boundaries specify the horizontal values of the knots of the CDF. Set axis = ‘quantile’ if you want to use distribution regression. If axis=’quantile’, the boundaries specify the vertical values of the knots of the CDF. |
Methods
cdf(pred,y,mask=None)
Returns the values of the CDFs evaluated at y.
Parameters:
Args |
Type |
Description |
---|---|---|
pred |
Tensor (float) |
Two-dimensional tensor to specify CDFs in which each row corresponds to a CDF. The size of the second column must be equal to len(boundaries)-1, and the sum of each row must be equal to one. |
y |
Tensor (float) |
Two-dimensional tensor to specify the values of CDFs to be evaluated. |
mask |
Tensor (bool) |
Optional. One-dimensional tensor to specify which rows should be evaluated at. |
Return type: Tensor (float)
icdf(pred,y,mask=None)
Returns the inverse cumulative density/mass function evaluated at value.
Parameters:
Args |
Type |
Description |
---|---|---|
pred |
Tensor (float) |
Two-dimensional tensor to specify CDFs in which each row corresponds to a CDF. The size of the second column must be equal to len(boundaries)-1, and the sum of each row must be equal to one. |
quantile |
Tensor (float) |
Two-dimensional tensor to specify the quantiles of CDFs to be evaluated. |
mask |
Tensor (bool) |
Optional. One-dimensional tensor to specify which rows should be evaluated at. |
Return type: Tensor (float)
Example 1
This code returns the values at 0.0, 1.0, and 2.0 of two CDFs illustrated in the figure.
The boundaries
specifies the boundaries of the knots of the CDFs.
The pred specifies the values of the knots of the CDFs, where the first and second rows correspond to the blue and red CDFs in the figure.
boundaries = torch.Tensor([0.0, 1.0, 2.0])
dist = dqs.torch.distribution.DistributionLinear(boundaries, axis='target')
pred = torch.Tensor([[0.7,0.3],[0.2,0.8]])
y = torch.Tensor([[0.0,1.0,2.0],[0.0,1.0,2.0]])
print(dist.cdf(pred,y))
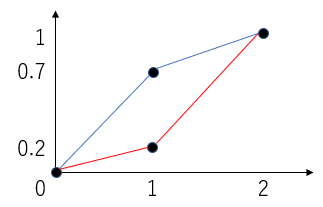
Output
[[0.0,0.4,1.0],[0.0,0.4,1.0]]
Example 2
You can change the axis by changing the parameter axis in the constructor. The blue CDF can be represented by using the following code.
boundaries = torch.Tensor([0.0, 0.7, 1.0])
dist = dqs.torch.distribution.DistributionLinear(boundaries, axis='quantile')
pred = torch.Tensor([[1.0,2.0]])
q = torch.Tensor([[0.0,0.7,1.0]])
print(dist.icdf(pred,q))
Output
[[0.0,1.0,2.0]]