How to use pmlayer.torch.layers.HLattice
In this tutorial, we demonstrate how to use pmlayer.torch.layers.HLattice
.
The source code used in this tutorial is available at github.
You can construct a model that consists of a single HLattice
layer by using the following code.
from pmlayer.torch.layers import HLattice
lattice_sizes = torch.tensor([4,4], dtype=torch.long)
model = HLattice(2,lattice_sizes,[0,1])
In this example, the first argument of HLattice
specifies that this model receives a two-dimensional input.
The second argument specifies that the granularity of lattice is 4 for both inputs.
The third argument specifies that the output value is monotonically increasing with respect to both of the input features.
We can train this model by using a standard training method for PyTorch models as shown in the following code.
# prepare data
a = np.linspace(0.0, 1.0, 10)
x1, x2 = np.meshgrid(a, a)
y = (x1*x1 + x2*x2) / 2.0
x = np.concatenate([x1.reshape(-1,1),x2.reshape(-1,1)], 1)
data_x = torch.from_numpy(x.astype(np.float32)).clone()
data_y = torch.from_numpy(y.reshape(-1,1).astype(np.float32)).clone()
# train model
loss_function = nn.MSELoss()
optimizer = torch.optim.SGD(model.parameters(), lr=0.001)
for epoch in range(5000):
pred_y = model(data_x)
loss = loss_function(pred_y, data_y)
model.zero_grad()
loss.backward()
optimizer.step()
By using the following code, you can see that the model is appropriately trained to learn the function \(f(x,y) = (x^2 + y^2)/2\).
# plot
pred_y_np = pred_y.to('cpu').detach().numpy().copy().reshape(x1.shape)
plt.figure(figsize=(4,3))
ax = plt.subplot(1, 1, 1)
im = ax.contourf(x1, x2, pred_y_np, levels=[0.0,0.2,0.4,0.6,0.8,1.0])
plt.subplots_adjust(left=0.1, bottom=0.1, right=0.7, top=0.9)
cax = plt.axes([0.8, 0.1, 0.05, 0.8])
plt.colorbar(im,cax=cax)
plt.show()
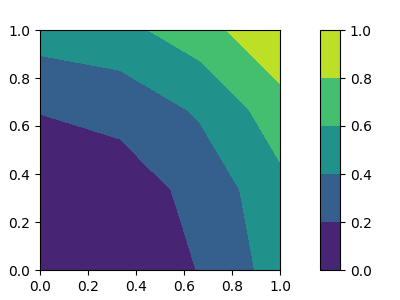
We note that this layer constructs a \(k \times k\) grid internally, where \(k \geq 2\) is the hyperparameter used to specify the granularity of the grid.
In this tutorial, we used \(k=4\) and the following figure shows the grid.
In the internal structure of HLattice
, each vertex of the grid is trained to learn the value \(f(x',y')\) of the input function \(f\), where \((x',y')\) is the coordinate of the vertex, while satisfying the monotonicity constraints.
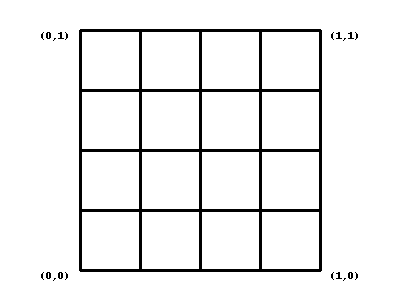